ESA Uses Drone Races to Develop AI for Space Missions
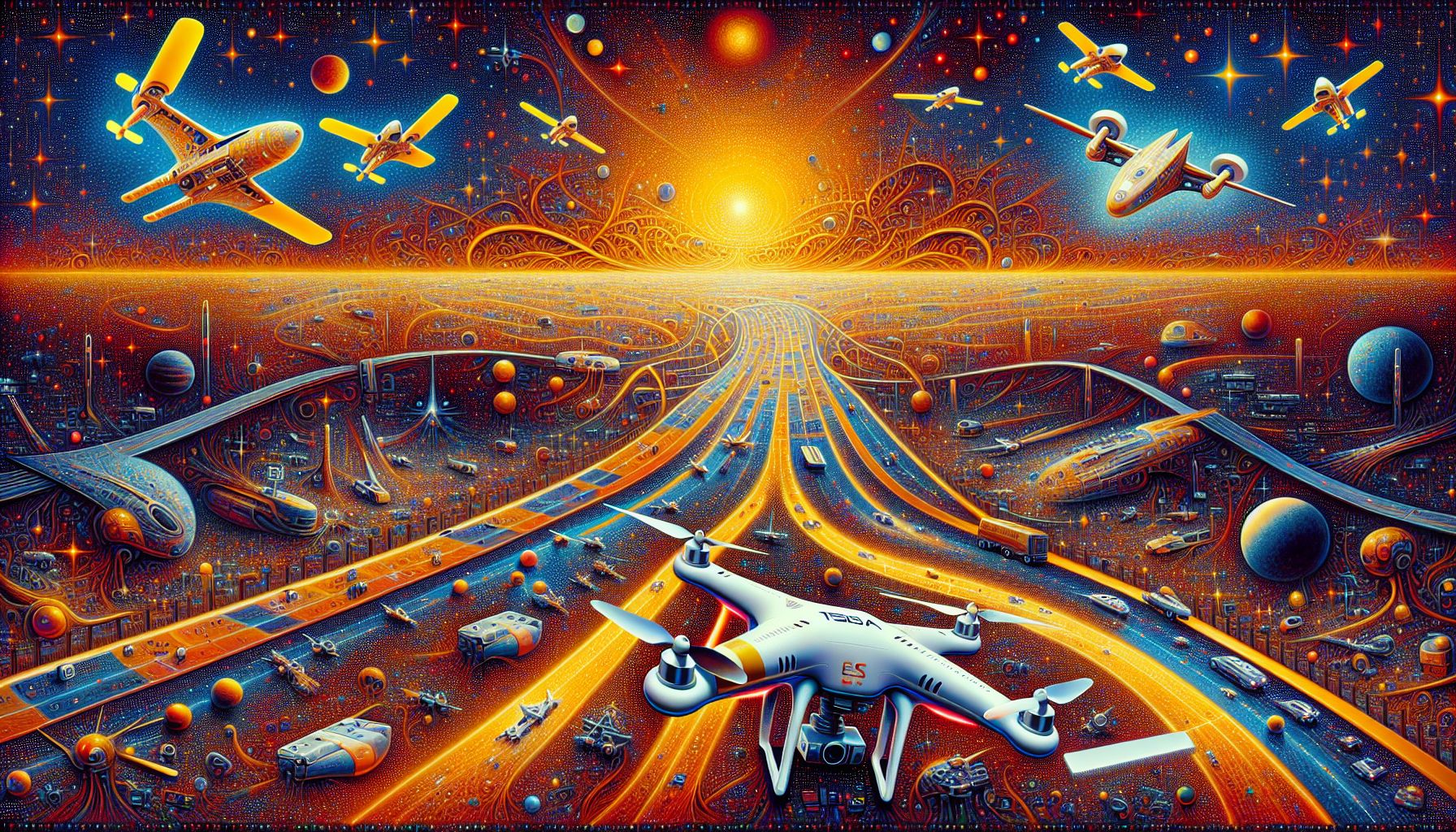
ESA and TU Delft are using drone races to test neural-network AI control systems for future space missions, aiming to enhance spacecraft autonomy and efficiency.
Testing in the ‘Cyber Zoo’
At the heart of this innovative research is the ‘Cyber Zoo’ at Delft University of Technology (TU Delft) in the Netherlands. Here, drones are raced against the clock to test the performance of neural-network-based AI control systems. This 10x10 meter test area serves as an ideal environment to evaluate the capabilities of these AI systems under real-world conditions. The drones navigate a set course, competing to achieve the best time, which provides valuable data on the AI’s performance and robustness.
Collaboration and Expertise
The project is a collaborative effort between the European Space Agency’s (ESA) Advanced Concepts Team (ACT) and TU Delft’s Micro Air Vehicle Laboratory (MAVLab). The research findings have been published in the latest issue of Science Robotics, showcasing the potential of neural networks for space missions. Dario Izzo, scientific coordinator of ESA’s ACT, emphasized the importance of this collaboration, noting that trainable neural networks could significantly enhance the autonomy of spacecraft by allowing them to handle complex maneuvers independently.
The Neural Network Approach
The neural network-based AI systems being tested are designed to optimize spacecraft operations by continuously replanning their trajectories. This approach, known as Guidance and Control Networks (G&CNETs), aims to improve mission efficiency and robustness. Unlike traditional methods where guidance is planned on the ground and control is executed by the spacecraft, G&CNETs enable the spacecraft to handle both tasks, making real-time adjustments as needed. This continuous replanning capability is particularly valuable in the unpredictable environment of space, where factors like gravitational variations and atmospheric turbulence can pose significant challenges.
Bridging the Reality Gap
One of the main challenges in implementing these neural networks is bridging the gap between simulation and real-world performance. In simulations, neural nets have shown promise when trained using ‘behavioral cloning,’ a method that involves mimicking expert behavior. However, real-world testing with drones is crucial to validate these simulations. Christophe De Wagter from TU Delft’s MAVLab noted that the dynamics of flying drones are much faster and noisier compared to spacecraft, making the tests in the Cyber Zoo an essential step in refining the neural network algorithms.
Implications for Future Space Missions
The implications of this research extend far beyond drone racing. By proving the effectiveness of neural-network-based AI systems in real-world scenarios, ESA and TU Delft are paving the way for more autonomous and efficient space missions. The ability of spacecraft to manage their own guidance and control without relying heavily on ground-based instructions could lead to more resilient and flexible missions. This innovation could be particularly beneficial for long-duration missions where real-time communication with Earth is limited.
Bronnen
- www.tudelft.nl
- www.esa.int
- www.esa.int
- www.aerospacetestinginternational.com
- www.spacewar.com
- www.copernical.com