AI Model Predicts Heart Failure Risk from Single ECG
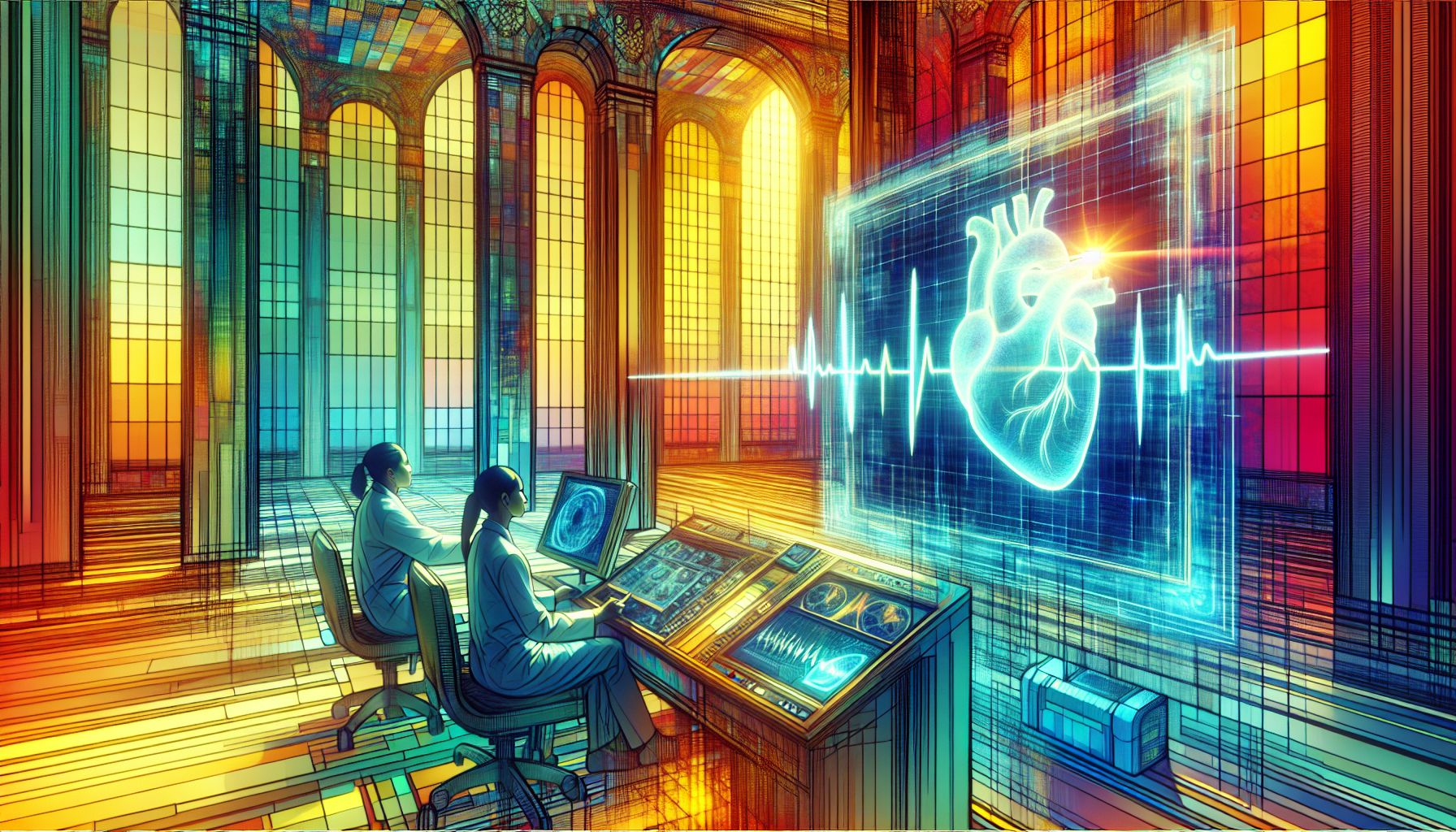
Researchers have developed an AI model that predicts heart failure risk from a single ECG, potentially transforming early detection and healthcare intervention.
Revolutionizing Heart Failure Detection
The development of this AI model marks a significant advancement in the field of cardiovascular health. By utilizing a single-lead electrocardiogram (ECG), the model can assess the risk of heart failure (HF) with remarkable accuracy. This innovation has the potential to revolutionize how early detection and healthcare interventions are approached, providing clinicians with a powerful tool to identify patients at risk well before symptoms manifest.
How the AI Model Works
The AI model, developed through a collaborative effort involving researchers from Yale New Haven Health System (YNHHS), the UK Biobank (UKB), and the Brazilian Longitudinal Study of Adult Health (ELSA-Brasil), uses a noise-adapted algorithm to analyze single-lead ECGs. The model was trained to identify left ventricular systolic dysfunction (LVSD), a key indicator of heart failure risk. By isolating lead I ECGs, the AI model could predict new-onset HF with high precision, outperforming traditional risk assessment methods such as the pooled cohort equations to prevent HF (PCP-HF) score.
Significant Findings
In the study, the AI model demonstrated impressive results across different cohorts. For instance, in the YNHHS cohort of 194,340 patients, a positive AI-ECG screen was associated with a 3- to 7-fold higher risk of HF. The model’s discrimination for new-onset HF was measured using Harrel’s C-statistic, yielding values of 0.725 in YNHHS, 0.792 in UKB, and 0.833 in ELSA-Brasil. Incorporating AI-ECG predictions alongside PCP-HF significantly improved risk prediction metrics, with an improvement in Harrel’s C-statistic (delta=0.112-0.114), an integrated discrimination improvement (IDI) of 0.078-0.238, and a net reclassification improvement (NRI) of 20.1%-48.8%.
Impact and Future Applications
This AI model presents a scalable and portable solution for heart failure risk stratification, making it feasible for use in wearable devices and large-scale community-based assessments. Dr. Amit Khera, one of the co-developers of the model and a leading researcher at the Yale School of Medicine, emphasized its potential: ‘Across multinational cohorts, a noise-adapted AI model with lead I ECGs as the sole input defined HF risk, representing a scalable portable and wearable device-based HF risk-stratification strategy.’ This innovation could lead to earlier interventions, reducing the incidence of heart failure and improving patient outcomes globally.
Supporting Research and Collaboration
The development and validation of this AI model were supported by multiple funding sources, including the National Heart, Lung, and Blood Institute and the Doris Duke Charitable Foundation. The research involved a multinational collaboration, highlighting the importance of global partnerships in advancing healthcare technology. Ensight-AI, co-founded by Dr. Khera and Mr. Sangha, played a crucial role in the creation of this model, showcasing the intersection of academia and industry in driving innovative solutions.
Ethical Considerations and Data Accessibility
The study adhered to all relevant ethical guidelines, with the Yale Institutional Review Board approving the protocol and waiving the need for informed consent due to the use of pre-existing data. The analyzed data from the UK Biobank cohort is available through the UK Biobank’s Access Management System, while data from ELSA-Brasil can be accessed upon reasonable request to the corresponding author. However, individual-level data for the YNHHS cohort cannot be made available due to HIPAA regulations.