AI Data Shortage Threatens Innovation: Study Reveals Looming Crisis
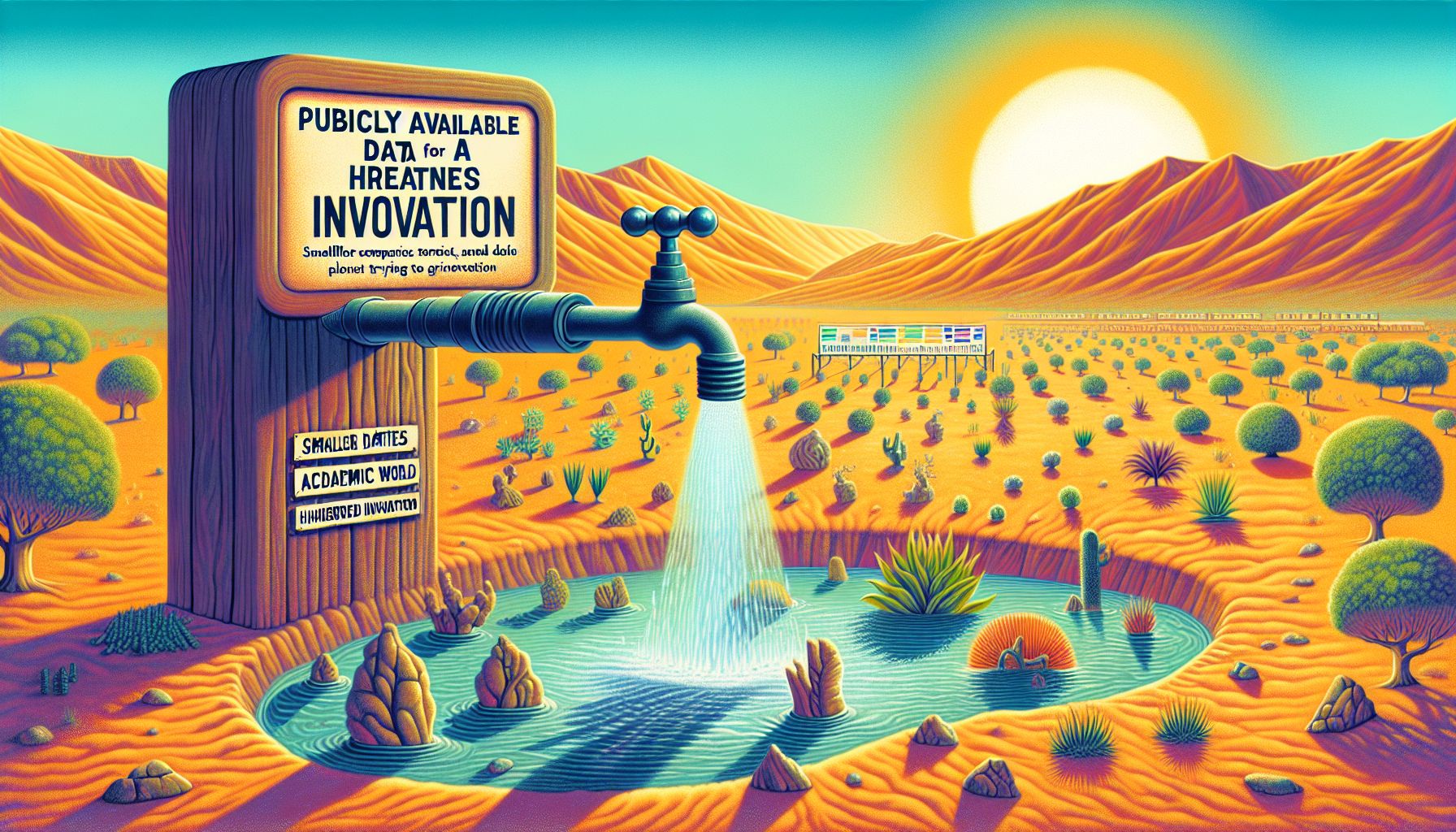
Amsterdam, Friday, 19 July 2024.
A recent study indicates that publicly available data for training AI is running low, posing significant challenges for AI development. This scarcity could hinder innovation, particularly affecting smaller AI companies and academic researchers who rely on open-source data. The situation may consolidate AI advancement in the hands of large corporations with substantial resources.
Implications of Data Scarcity for AI Development
The drying up of public data sources has far-reaching implications for the AI industry. A significant portion of AI development relies on vast datasets to train models for tasks such as language processing, image recognition, and predictive analytics. With 5% of all data and 25% of high-quality data sources now restricted[1], smaller companies and researchers face increasing difficulties in accessing the necessary data. This could lead to a slowdown in innovation as these entities struggle to develop and refine AI technologies.
Challenges for Smaller AI Companies and Academia
The restricted access to data disproportionately affects smaller AI firms and academic institutions. Unlike major corporations, these smaller entities often lack the financial resources to negotiate data access deals. Companies such as Reddit, The Associated Press, and News Corp. have started monetizing their data, making it exclusive to paying AI firms[1]. This commercialization of data can stifle the progress of startups and academic researchers who depend on freely available data for their projects.
Potential Solutions to Data Scarcity
In response to the data scarcity challenge, several innovative solutions are being explored. One approach is the generation of synthetic data, which aims to replicate real-world data without the associated access restrictions[2]. Companies like Nvidia are also developing platforms such as DRIVE Sim to create simulated environments for training autonomous vehicle systems[2]. Another strategy, federated learning, allows AI models to be trained across multiple institutions without the direct sharing of sensitive data, thereby preserving privacy while still enabling collaborative learning[2].
Economic and Infrastructure Impacts
The data scarcity issue is part of a larger context of AI infrastructure demands. Goldman Sachs estimates that $1 trillion will be spent on AI-related infrastructure, including data centers and semiconductors, in the coming years[3]. This massive investment underscores the importance of data in driving AI advancements. However, the financial returns on these investments remain uncertain, especially if data scarcity continues to impede the development of breakthrough applications[3].
Future Outlook: Efficiency and Innovation
As the landscape of AI development shifts, there is a growing emphasis on making AI models more efficient with limited data. Researchers are focusing on techniques like few-shot learning, transfer learning, and unsupervised learning to maximize the utility of available data[2]. Additionally, there is an increasing push towards explainable AI models and robust data curation practices to ensure the quality and reliability of AI systems[2]. These efforts aim to sustain innovation in the face of data shortages and maintain the momentum of AI advancements.