New Method Developed to Prevent AI Hallucinations
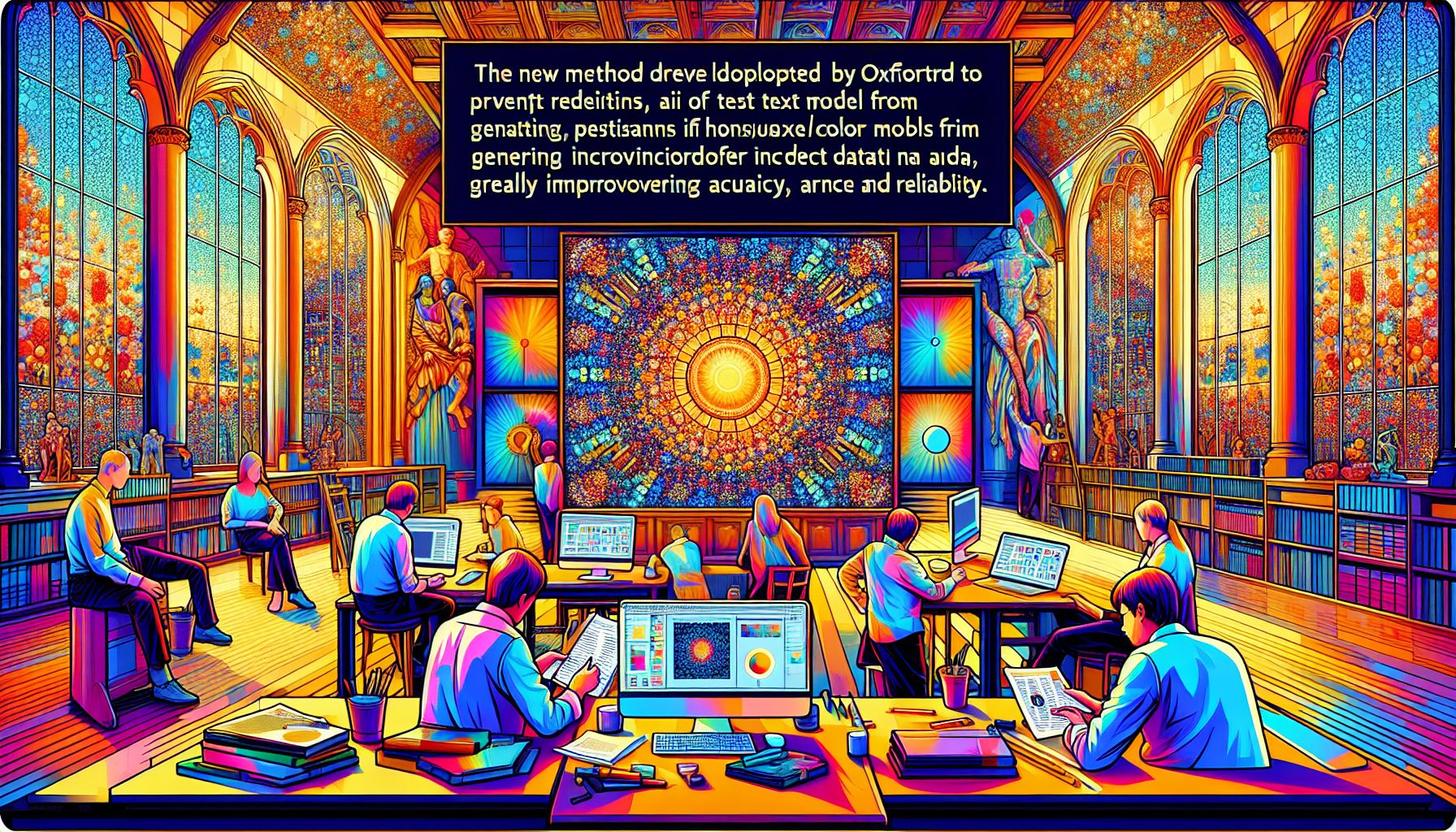
Oxford researchers have created a technique to predict and prevent AI text models from generating false information, enhancing accuracy and reliability across various applications.
Understanding AI Hallucinations
AI hallucinations occur when generative models produce information that is not grounded in reality. These hallucinations can range from slight inaccuracies to completely fabricated data, posing significant risks in critical fields such as healthcare, finance, and law [1]. The phenomenon is particularly concerning in applications where incorrect information can lead to severe consequences, such as medical misdiagnoses or legal misjudgments [2].
The Innovation: Semantic Entropy
The new method developed by Oxford researchers focuses on measuring semantic entropy in AI responses. Semantic entropy indicates the level of uncertainty in the generated content, distinguishing between uncertainties in meaning and wording [1]. Lower entropy suggests high confidence in the response, while higher entropy points to potential inaccuracies. By identifying these uncertainties, the method can predict when an AI is likely to hallucinate, allowing for preemptive corrections.
Applications and Effectiveness
This method has been tested on six large language models (LLMs), including GPT-4 and LLaMA 2, demonstrating its effectiveness in identifying false answers across various domains such as Google searches, biomedical queries, and mathematical problems [1]. The ability to spot inaccuracies in these diverse fields highlights the method’s versatility and potential for broad application. This development is crucial as generative AI continues to be integrated into more sectors, necessitating reliable and accurate outputs.
Trade-offs and Challenges
While the new method significantly improves the reliability of AI-generated content, it does come with increased computational costs. As noted by Professor Yarin Gal, the trade-off between cost and reliability is a critical consideration. ‘Getting answers from LLMs is cheap, but reliability is the biggest bottleneck. In situations where reliability matters, computing semantic uncertainty is a small price to pay,’ Gal explained [1]. This highlights the need for a balanced approach in implementing the method, ensuring that the benefits outweigh the additional resources required.
Broader Implications
The implications of this innovation extend beyond academia and into practical applications. For instance, Google’s recent issues with its AI Overview feature, which was disabled due to misleading answers, underscore the importance of reliable AI systems [1]. The method developed by Oxford researchers could provide a solution to such problems, enhancing the trustworthiness of AI in public-facing applications. Furthermore, this technique could be adapted to other generative AI models, reducing the prevalence of hallucinations and improving overall accuracy.