Advancements in Explainable AI Enhance Transparency in AI Models
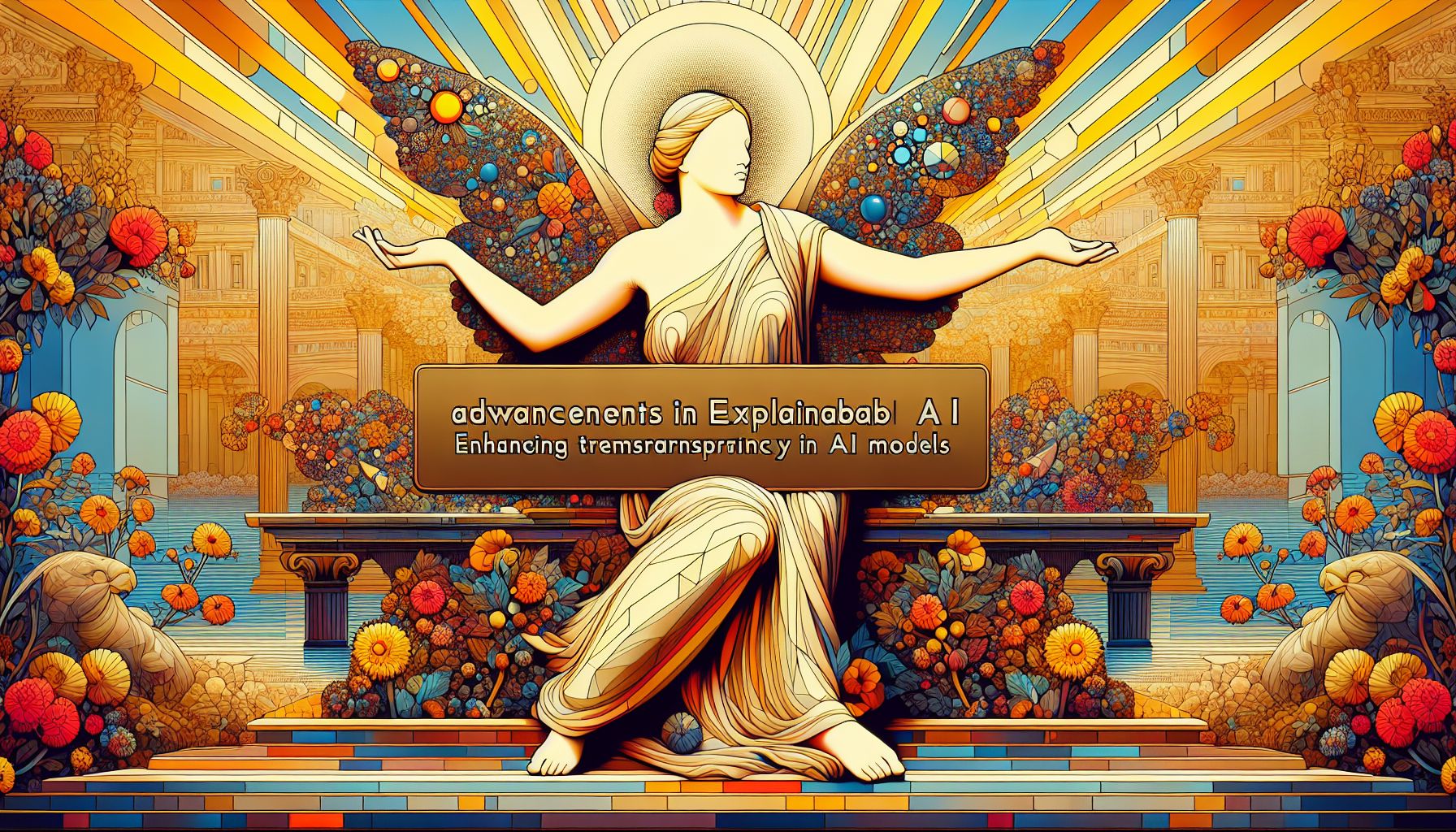
New developments in Explainable AI (XAI) are making AI models more transparent and comprehensible, crucial for ethical AI deployment and ensuring trust in AI applications.
The Importance of Explainability in AI
In the contemporary landscape of AI, the opaqueness of ‘black-box’ models has raised significant concerns, especially in high-stakes domains such as healthcare, finance, and autonomous driving. These concerns are rooted in the inability of users and stakeholders to understand and trust the decisions made by AI systems. Explainable AI (XAI) addresses these issues by providing a framework that makes AI’s decision-making processes transparent and comprehensible. This is not just a technical necessity but a moral imperative, ensuring that AI systems can be held accountable and their decisions can be scrutinized and justified.
Key Players and Innovations
Several organizations and researchers are at the forefront of XAI advancements. The IEEE’s comprehensive review on XAI highlights the state-of-the-art methods and applications that are transforming opaque machine learning outputs into understandable decisions. Methods such as LIME (Local Interpretable Model-agnostic Explanations) and SHAP (SHapley Additive exPlanations) are pivotal in this transformation[1]. Additionally, companies like Aporia are developing ML observability solutions that enhance the transparency of AI models, ensuring that stakeholders can understand and trust model predictions[2].
Practical Applications and Benefits
The practical applications of XAI span various industries. For instance, in healthcare, XAI can help clinicians understand the rationale behind AI-based diagnostic tools, leading to more informed decision-making. In finance, it assists in explaining credit scoring algorithms to both regulators and customers, ensuring fairness and compliance with regulations such as the EU’s GDPR[2]. Additionally, XAI plays a crucial role in debugging and improving AI models by identifying biases and errors that were previously hidden within complex structures[1].
Challenges and Future Directions
Despite its potential, XAI faces several challenges, including the trade-off between model performance and interpretability. Simplifying a model to make it more explainable can sometimes reduce its accuracy. Moreover, there are ongoing debates about the best methods to achieve explainability without compromising the confidentiality and complexity of proprietary algorithms. Future research is focused on developing more robust and comprehensive XAI techniques that can balance these trade-offs effectively. The IDA symposium at Stockholm University, which emphasized innovative ideas in AI, highlighted the importance of ethical AI and the need for continuous improvement in explainability methods[3].
Conclusion
The advancements in Explainable AI signify a critical step towards more transparent and accountable AI systems. By bridging the gap between complex AI mechanisms and human understanding, XAI not only fosters trust but also aligns AI applications with ethical standards. As research and development in this field continue, the future of AI looks promising with more interpretable and reliable models leading the way.