TU Eindhoven Pioneers On-Chip AI Training
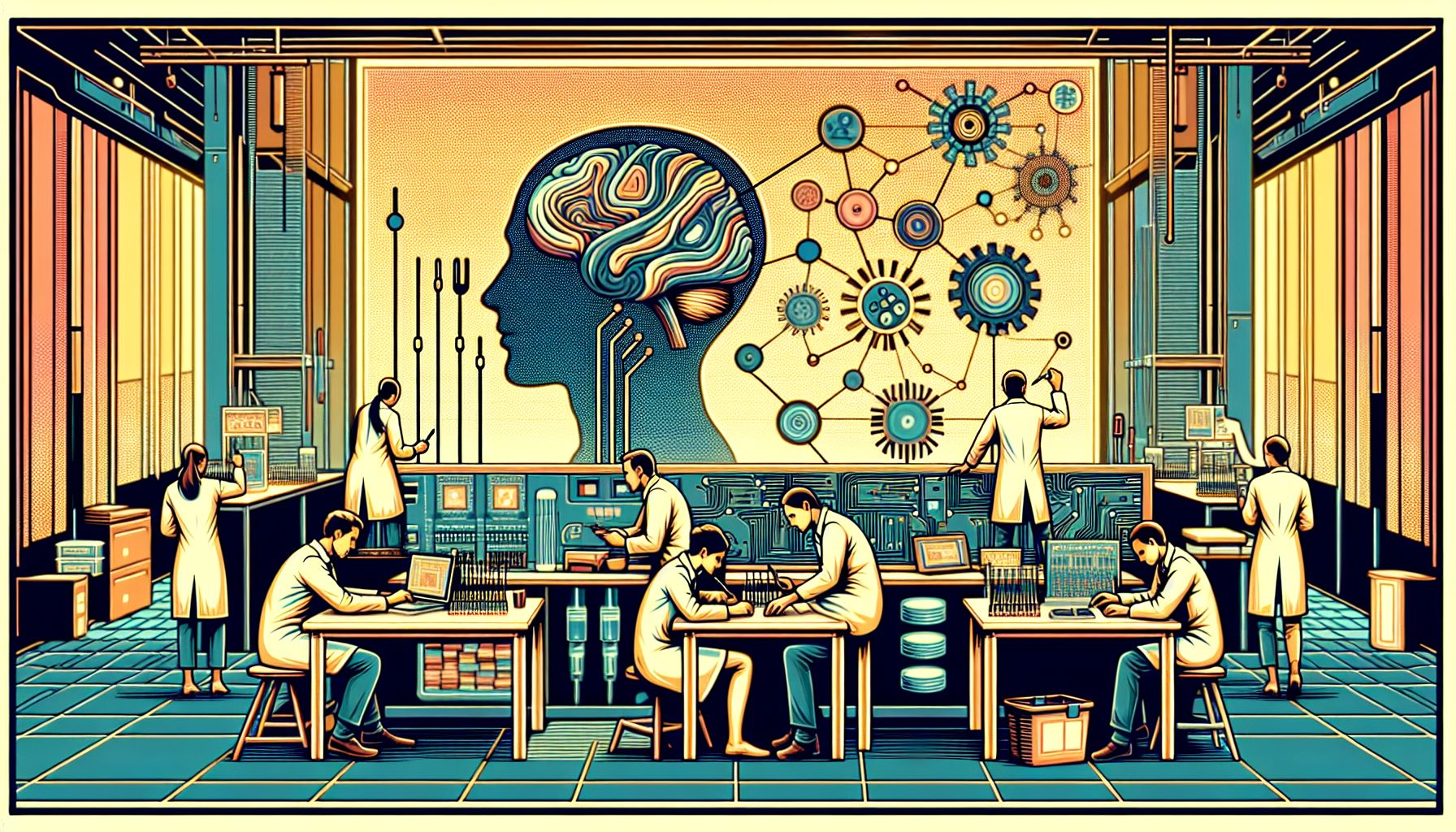
Eindhoven, Monday, 15 July 2024.
Researchers at TU Eindhoven have developed a groundbreaking method for training AI models directly on neuromorphic chips, mimicking brain-like functions. This innovation could lead to more efficient and energy-saving AI chips, potentially revolutionizing the field of artificial intelligence.
Introduction to Neuromorphic Chips
Neuromorphic chips represent a significant advancement in the semiconductor industry, particularly in the realm of AI and machine learning. By replicating the way the human brain processes information through electrical charges between neurons, these chips aim to bring a higher level of efficiency and functionality to AI training. Traditional AI training methods are often cumbersome, requiring models to be trained on computers before being transferred to chips, which consumes considerable time and energy.
The Innovation at TU Eindhoven
Researchers at Eindhoven University of Technology (TU/e), led by Yoeri van de Burgt and Marco Fattori, have developed a neuromorphic device capable of on-chip training. This method eliminates the need to transfer pre-trained models to the chip, thereby addressing the inefficiencies associated with conventional training methods. Van de Burgt, an associate professor in the Mechanical Engineering faculty, and Fattori from the Electrical Engineering department, have created a two-layer neural network using electrochemical random-access memory (EC-RAM) components made of organic materials.
How It Works
The neuromorphic device developed by the TU/e team leverages memristors—memory resistors that ‘remember’ the amount of electrical charge that has flowed through them. This capability is crucial as it allows the chip to store and process information in a manner similar to the human brain. However, the stochastic nature of memristors has been a challenge, as they need to be programmed individually and checked for errors. Van de Burgt and Fattori’s circuit design circumvents these limitations by integrating EC-RAM components, which enable more reliable and scalable neural networks.
Benefits and Future Prospects
The primary advantage of this innovation is the significant reduction in energy consumption and time required for AI model training. By training AI models directly on the hardware, the process becomes more streamlined and efficient. This breakthrough could lead to the development of AI chips that are not only more powerful but also more sustainable. However, for practical applications, the current two-layer network needs to be expanded. Comparatively, large language models like GPT-4 and Llama 3 have 120 and 80 layers, respectively. The next steps involve adding more layers to the network and collaborating with industry partners to test the technology on real-life data problems.
Collaborative Efforts and Industry Impact
The work by Van de Burgt and Fattori is part of a larger research initiative involving Tim Stevens and Eveline van Doremaele. Published in the journal Science Advances, this research is a testament to the collaborative efforts aimed at advancing semiconductor technology. TU Eindhoven’s partnership with institutions like KU Leuven further strengthens the semiconductor knowledge and innovation ecosystems in Europe. This collaboration is aligned with the European Chips Act and aims to position the Brainport and Mindgate regions as pivotal players in the global semiconductor landscape.