Breakthrough in Neural Network Training: TU/e Researchers Simplify AI Chip Development
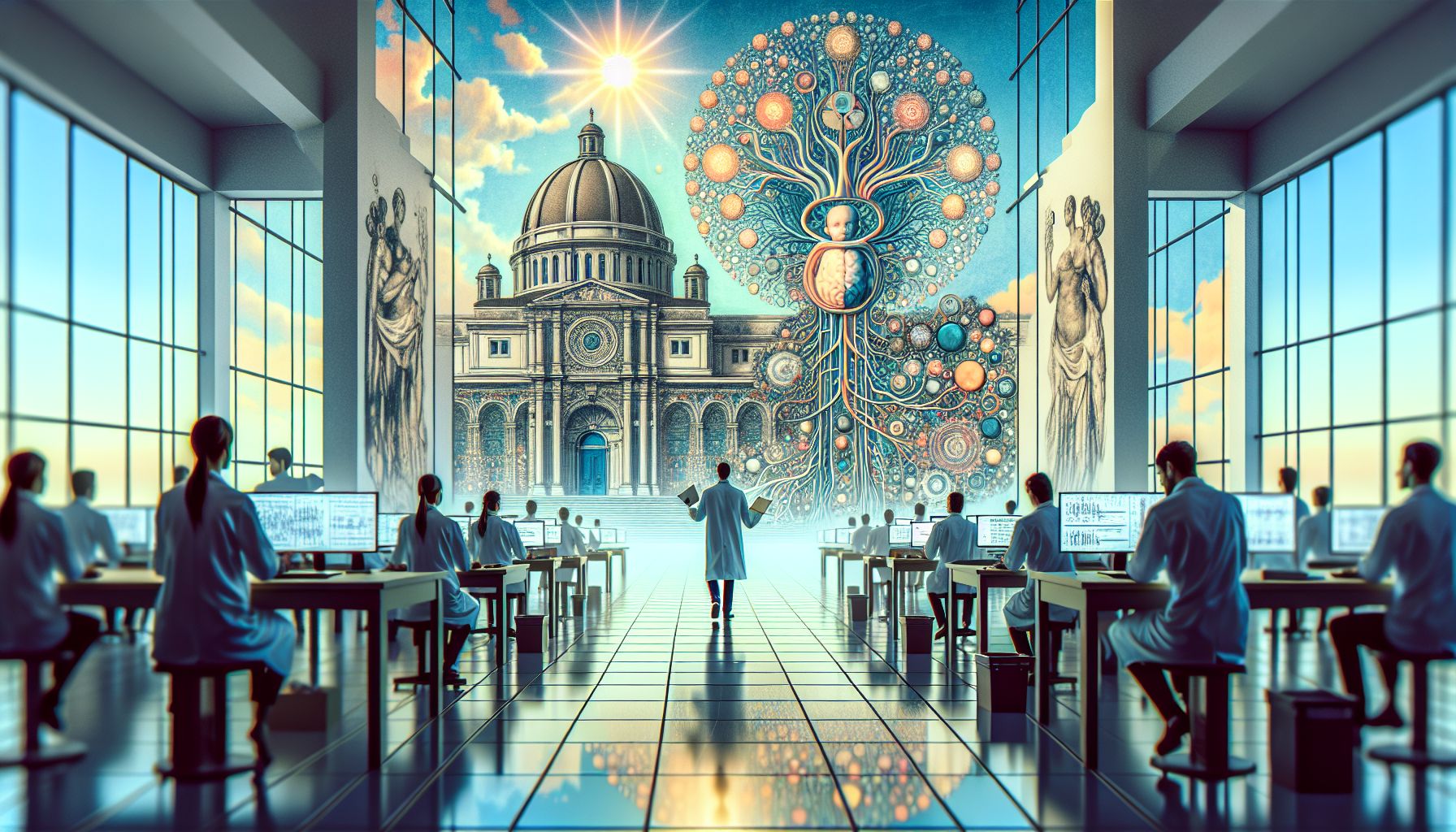
Eindhoven, Monday, 29 July 2024.
Eindhoven University of Technology researchers have revolutionized neuromorphic chip training, making neural network implementation more efficient. Their innovative approach, published in Science Advances, enables on-chip training, potentially transforming AI applications across various industries.
Understanding Neuromorphic Chips
Neuromorphic chips are designed to replicate the brain’s neuron firing process using specialized components known as memristors. These memristors can ‘remember’ the amount of electrical charge that has flowed through them, making them an ideal candidate for mimicking the brain’s neural networks. However, the traditional methods of training these chips, whether on a computer or in-situ, have been both time-consuming and energy-intensive.
The Innovation by TU/e Researchers
Led by Associate Professor Yoeri van de Burgt and Marco Fattori, a team from Eindhoven University of Technology (TU/e) has successfully developed a method for on-chip training. This breakthrough eliminates the need to transfer trained models from external computers, significantly cutting down on energy costs and training time. The research, detailed in the paper ‘Hardware implementation of backpropagation using progressive gradient descent for in situ training of multilayer neural networks,’ showcases a new technique that allows for efficient training directly on the neuromorphic hardware.
How It Works
The team integrated electrochemical random-access memory (EC-RAM) components into a two-layer neural network. This integration allows the neural network to perform training directly on the chip. EC-RAM components facilitate the progressive gradient descent method, a technique that optimizes the network by continuously adjusting the weights of the nodes, thereby improving accuracy and efficiency. This method not only accelerates the training process but also makes it more energy-efficient.
Benefits and Future Applications
The ability to train neural networks directly on neuromorphic chips has numerous advantages. It reduces energy consumption, speeds up the training process, and eliminates the need for extensive data transfers. This innovation has potential applications across various fields, including transport, communication, and healthcare. As neural networks are integral to solving complex problems with large amounts of data, this breakthrough could lead to more efficient AI systems capable of tackling real-world challenges.
Industry Collaboration and Future Goals
The TU/e team aims to collaborate with industry partners to scale up this technology for practical applications. ‘My dream is for such technologies to become the norm in AI applications in the future,’ stated Yoeri van de Burgt. By working with industry, the researchers hope to integrate their on-chip training methods into commercial AI systems, further enhancing their efficiency and adaptability.