TU Delft Uses Neural Networks to Address GRACE Data Gaps
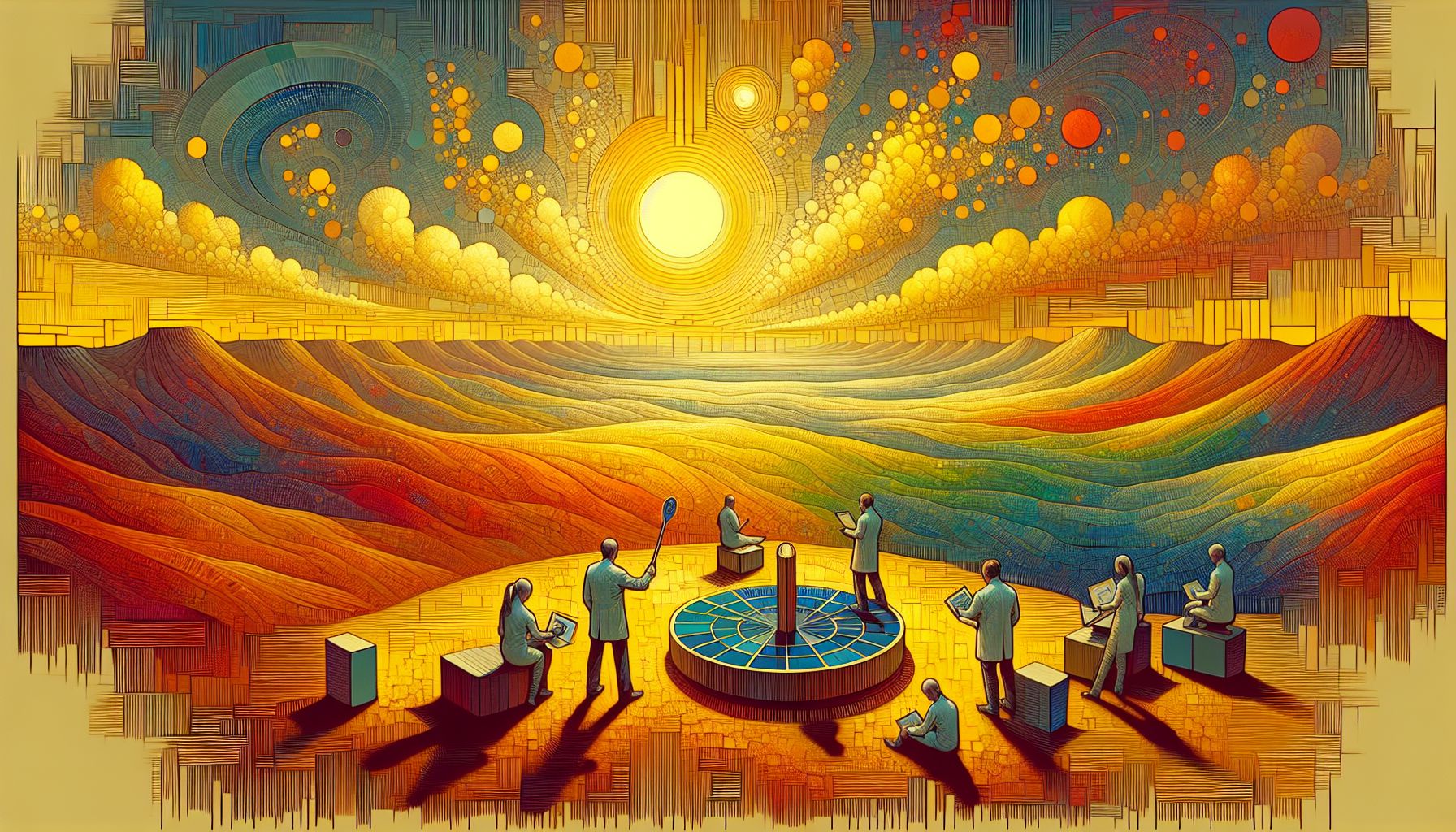
Delft, Friday, 10 January 2025.
Researchers at TU Delft are using neural networks to fill data gaps in GRACE mission datasets, significantly enhancing climate modeling accuracy and understanding of Earth’s water cycles.
Innovative Solution for Climate Data
The research team at TU Delft has developed a sophisticated neural network approach to address a critical challenge in climate science: gaps in data from NASA’s GRACE (Gravity Recovery and Climate Experiment) mission [1]. Launched in 2002, GRACE has been instrumental in monitoring Earth’s gravity field and tracking changes in equivalent water height (EWH), but data gaps have historically hindered accurate climate modeling [1][4]. The neural networks are specifically trained using ESA’s Swarm EWH data and NASA’s GLDAS soil moisture data to predict GRACE EWH measurements [1].
Proven Performance and Accuracy
The effectiveness of this innovative approach has been demonstrated through extensive testing, involving 20,400 models across major river basins [1]. The results show that the neural network solutions achieve significantly lower errors in EWH gravity field measurements compared to existing Swarm data [1]. This breakthrough in data processing enhances our ability to track mass redistribution across Earth’s surface, providing crucial insights for climate change research [1][4].
Impact on Climate Science
The implementation of this new data processing technique, which began in late 2024 [4], represents a significant advancement in climate science methodology. By incorporating noise-level variation and additional training data, the research has shown substantial reductions in both errors and uncertainties [1]. This improvement in data quality and consistency is particularly valuable for climate scientists working to understand and predict global water cycle changes [4].
Future Implications
As climate modeling becomes increasingly critical for understanding global environmental changes, this advancement in data processing represents a significant step forward in climate science capabilities [4]. The research demonstrates the potential of machine learning techniques to enhance our understanding of Earth’s systems, with continued improvements expected as the methodology evolves [4]. This innovation positions TU Delft at the forefront of climate data analysis, contributing to more accurate climate change predictions and better understanding of global water resources [1].