Analog AI Computers: The Next Frontier in Artificial Intelligence
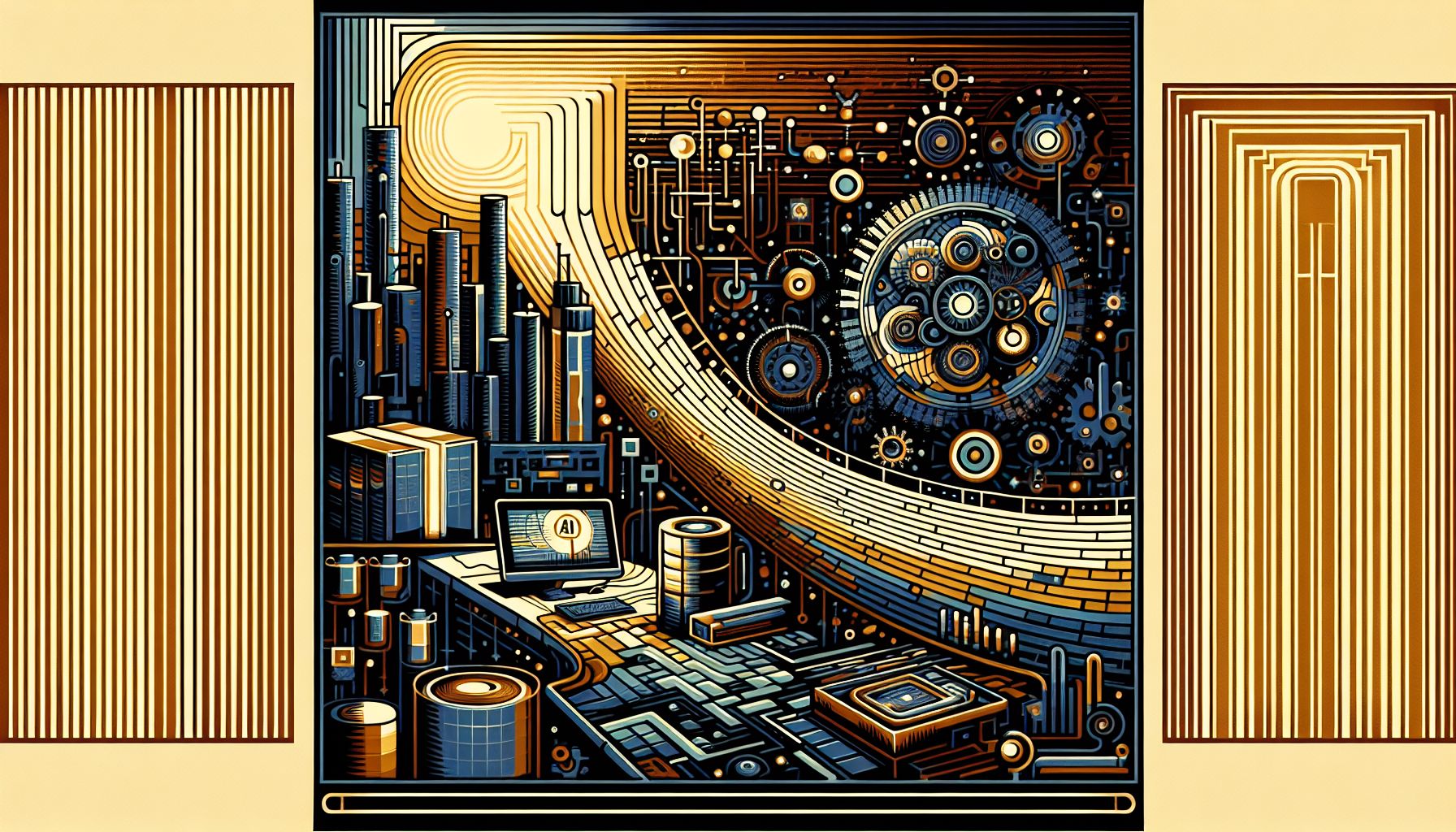
Amsterdam, Thursday, 15 August 2024.
Emerging research suggests analog AI computers could revolutionize artificial intelligence, offering improved efficiency and power. This shift from digital to analog computing promises faster AI training, enhanced energy efficiency, and more responsive real-time applications, potentially transforming the future of AI technology.
The Case for Analog AI
Digital computers, which form the backbone of current AI systems, face significant challenges in keeping up with the increasing complexity of AI models. Issues such as slower training speeds, suboptimal performance, and higher energy consumption are becoming pronounced. Traditional digital systems, based on the Von Neumann architecture, separate memory and processing tasks, causing bottlenecks that reduce both processing speed and energy efficiency[1].
IBM’s Pioneering Efforts
IBM has been at the forefront of developing analog AI technology, introducing a 14-nanometer analog AI chip with 35 million memory cells. This chip uses ‘compute-in-memory’ and ‘phase-change memory’ technology, which processes data within the memory itself, significantly reducing energy overheads and speeding up computations. According to IBM, this chip performed AI tasks seven times faster and with a fourteenfold increase in energy efficiency compared to traditional hardware[1].
How Analog AI Works
Analog AI, also known as neuromorphic or brain-inspired computing, mimics the structure of biological neural networks using continuous signals rather than discrete binary values. This approach allows for parallel processing, enabling the system to perform multiple calculations simultaneously. This parallelism significantly enhances efficiency, particularly for complex tasks such as real-time speech recognition and computer vision[1].
Environmental and Performance Benefits
One of the most compelling advantages of analog AI is its potential for improved energy efficiency. Digital computers consume vast amounts of energy for complex AI calculations, which raises operational costs and contributes to a larger CO2 footprint for data centers. Analog AI systems, by processing data continuously and reducing data conversion overheads, offer substantial energy savings, making them more environmentally friendly. Additionally, the improved speed and efficiency can lead to faster AI training and more responsive real-time applications[1].
Broader Implications and Future Prospects
The transition to analog AI could have far-reaching implications for various industries. For example, in robotics and the Internet of Things (IoT), the enhanced processing capabilities and energy efficiency could lead to more advanced and autonomous systems. Companies like Analog Devices are already demonstrating the potential of edge AI with their MAX78000 microcontroller, which enables AI inferences directly next to camera sensors, reducing data transmission and latency[5]. As research progresses and more companies invest in this technology, analog AI could very well become the standard for future AI systems, driving innovation and efficiency across multiple sectors.