TU Delft Drone Racing Advances AI for Space Missions
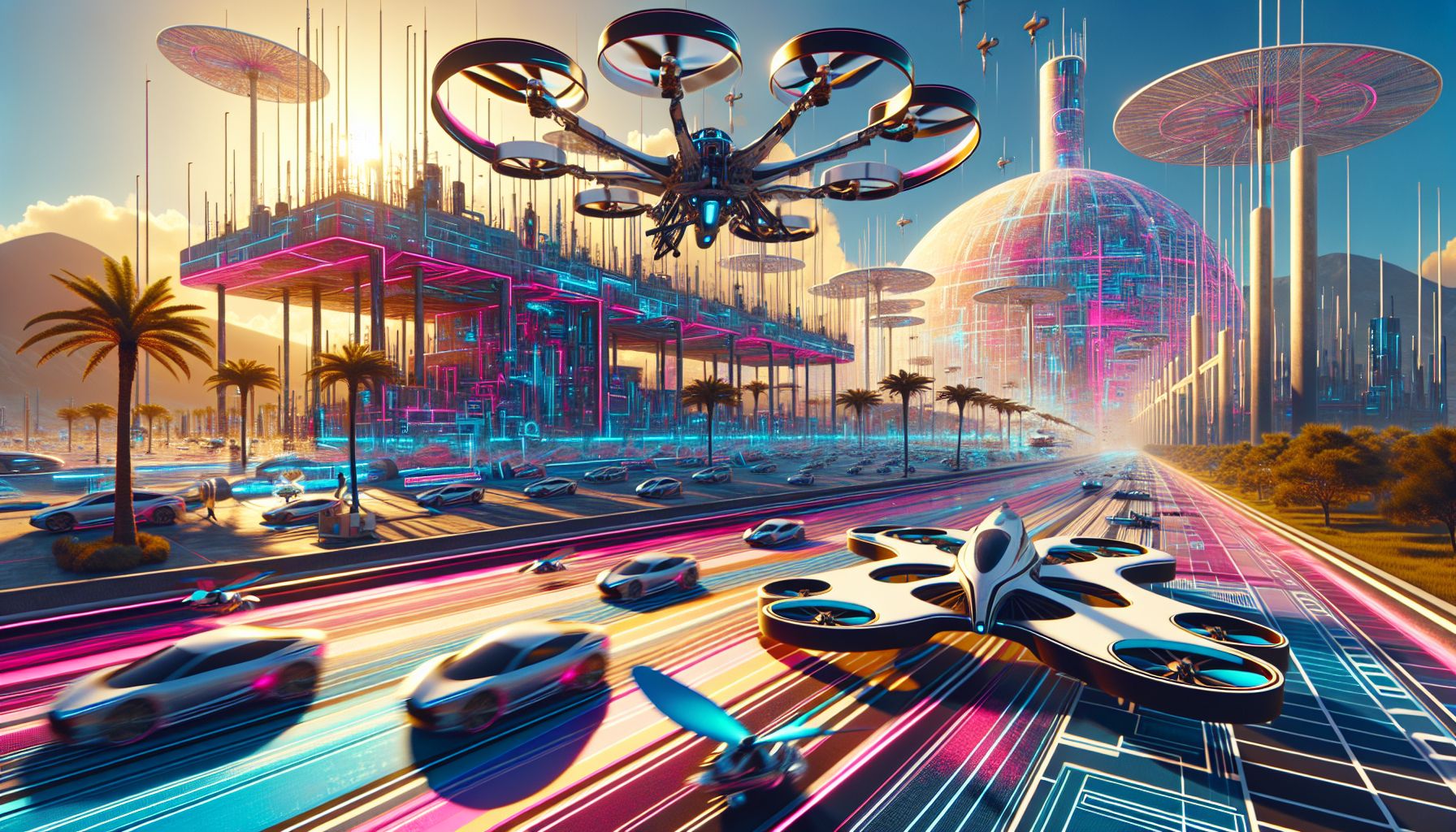
Delft, Sunday, 22 December 2024.
TU Delft’s Cyber Zoo uses drone racing to test AI systems for space missions, showcasing potential for improved spacecraft control and efficiency in neural network applications.
Neural Networks in Space Control
TU Delft’s MAVLab, in collaboration with ESA’s Advanced Concepts Team, is pioneering the use of neural-network-based AI control systems for future space missions [1]. The research, conducted at TU Delft’s Cyber Zoo, involves testing drones against the clock to evaluate AI performance [1]. This innovative approach utilizes G&C Nets, allowing spacecraft to continuously replan trajectories based on current positions, marking a significant advancement in space navigation efficiency [1].
Real-World Testing and Validation
The testing environment pairs human-steered quadcopters with autonomous drones using trained neural networks to navigate racing courses [1]. This approach represents a departure from traditional spacecraft maneuver planning, which typically relies on pre-planned trajectories uploaded from Earth [1]. The system demonstrates remarkable efficiency, operating with modest computational resources of around 30,000 parameters and less than 360 neurons [1].
Bridging Simulation and Reality
A key challenge in the research has been addressing the ‘reality gap’ between simulated and actual drone performance [1]. The team has developed innovative solutions by identifying discrepancies during flight and teaching the neural network to adapt accordingly [1]. This approach has proven crucial for establishing trust in AI systems for space applications, as the team works to validate performance in real-world conditions [1].
Future Space Applications
The research demonstrates significant potential for advancing spacecraft control systems [1]. By testing these systems in the controlled environment of drone racing, researchers can evaluate their performance under time-sensitive conditions that mirror the challenges of space operations [1]. The successful implementation of these neural network-based control systems in drone racing provides a foundation for their eventual deployment in space missions, though specific mission dates remain under development [1].